In the previous post I introduced the tiny spell checker challenge and some of the solutions our team discussed. In this post I'll go into a bit more detail about the solution I put forward, how well it performed, and some limitations of the approach.
I combined the use of a bloom filter with additional validation using n-grams.
Bloom Filter
The bloom filter is a well-known data structure for checking the existence of an element in a set. The bloom filter provides good space efficiency at the cost of a probabilistic result. In other words, you can ask whether an item exists within a set and get one of two answers: no or probably. You can control how often probably is returned for an item that is not in the set through a few parameters: the number of items in the filter, the number of bits in the filter, and the number of hash functions used to compute the bit indices.
In my case, the input size was fixed (the number of words in the dictionary) so I could control the number of bits and the number of hashes. Since this challenge was primarily about size I fixed the number of hashes and experimented with a bit count that would fit within the challenge constraints.
That left me with a calculated probability of returning a false positive somewhere around 1 in 3. We will see how this ultimately impacts the performance of the spell checker below. However, there was still a bit of space remaining to attempt to reduce the number of false positives so I looked to using something else I was exploring in parallel: n-grams.
N-gram Validation
I spent a significant amount of time while exploring the solution thinking about compression. How could I compress the 6.6M to something even close to the 256K size limit for the challenge (zip gets close to 1.7M, for example). As part of that I looked into n-gram substitution - specifically, could I take advantage of known suffixes or prefixes to reduce the amount that needs to otherwise be compressed.
This ultimately didn't pan out as I ended up needing to store more in metadata to decode the table than the size reduction of the approach. However, one of the challenges with bloom filters is false positives, especially when the table size is constrained and there are many inputs. So when I pivoted to using a bloom filter I returned to the n-gram approach as as a secondary validation mechanism.
For each of the words in the dictionary I extracted each n-gram and added it to a set. Checking for a valid word then consisted of extracting each n-gram of the input word and verifying it was part of the set.
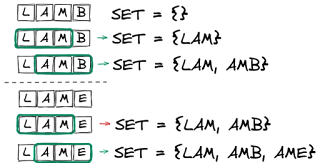
Much like the bloom filter this has the property of no false negatives (i.e. any n-gram not in the set must be from a misspelled word) with the potential for false positives (i.e. can construct a word from the n-grams set that is not a valid word from the original dictionary).
For the 6.6M dictionary, the following table indicates the space necessary to encode all of the n-grams of a particular size.
N | Size |
2 | 1,766 |
3 | 33,892 |
4 | 330,872 |
5 | 1,459,024 |
To keep within the size constrains of the problem I could only use n-grams of size 2 or 3. I wanted the larger since this was for validation and I felt there would be higher false positive rate using the shorter n-gram size (although, to be fair, I did not measure this).
Building Metadata into the Executable
As I wanted an all-in-one solution, I serialized both the bloom filter and n-gram set to disk as a separate process before building the executable.
I used a std::bitset for the bloom filter and made sure to set the size to something divisible by 8 so it was possible to serialize without accounting for padded bits. For the n-gram set I ended up using std::set< std::string > so serialization was just a matter of writing the 3-byte sequences out to file. The order in the set didn't matter so I could simply dump the values contiguously given I knew the n-gram size.
With both data sets serialized to disk I used the linker to generate object files out of the binary data so I could link them when building the final executable.
$ ld -r -b binary -o ngram_data.o ngram.bin
$ nm ngram_data.o
0000000000008464 D _binary_ngram_bin_end
0000000000008464 A _binary_ngram_bin_size
0000000000000000 D _binary_ngram_bin_start
Limitations
Capitalization: To achieve some of the size results I had to make some concessions. For example, all string operations (hashing and n-gram) is performed on lower case strings. This completely removes any capitalization support in this application (a necessary feature for any serious spell checker).
Speed: Because of the way the dictionary is packed, there is a large upfront cost (relative to the check itself) to load the information into usable data structures before a single word can be checked. I would have liked to have had something that could be searched directly from memory but opted for a more simplistic serialization approach instead.
Results
I computed the full dictionary (6.6M) for n-grams of size 3, hashed each of the 654K words for the bloom filter, and serialized and linked each of those into an executable that came in at 243K.
In the worst test case (replacing 50% of the dictionary words with invalid words) the f-score was about 0.86 without using n-grams and closer to 0.88 with n-gram validation.
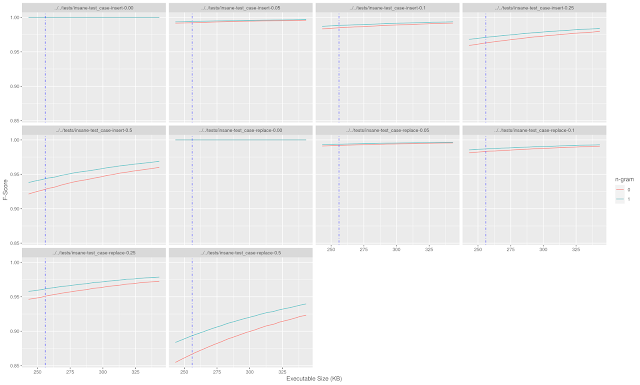
Looking back at the expected false positive rate of the bloom filter (roughly 1 in 3) this is the expected output. With a 654K input, 50% of which are replaced with invalid words, we would expect somewhere around 115K false positives which is consistent with an f-score near 0.85.
Adding in the n-gram effectively helps lower the probability of a false positive from 1 in 3 to just over 1 in 4. There is plenty of room here for optimizations such as fine-tuning the bloom filter to be closer to the size limit, pruning the n-gram list to the most frequent values to reduce size, and so on, but this was a decent start to thinking about the problem.